Generative AI in Business: Transforming Industries with Synthetic Data
The rise of synthetic data generation is fundamentally reshaping how enterprises use AI. Synthetic datasets – generated by AI rather than collected from real-world sources – solve critical data challenges in business. Recent research highlights this surge: the global synthetic data generation market will explode from about $324 million in 2023 to $3.7 billion by 2030 (a 41.8% CAGR). This growth is driven by the need for high-quality, diverse training data where real data are scarce, sensitive, or expensive.
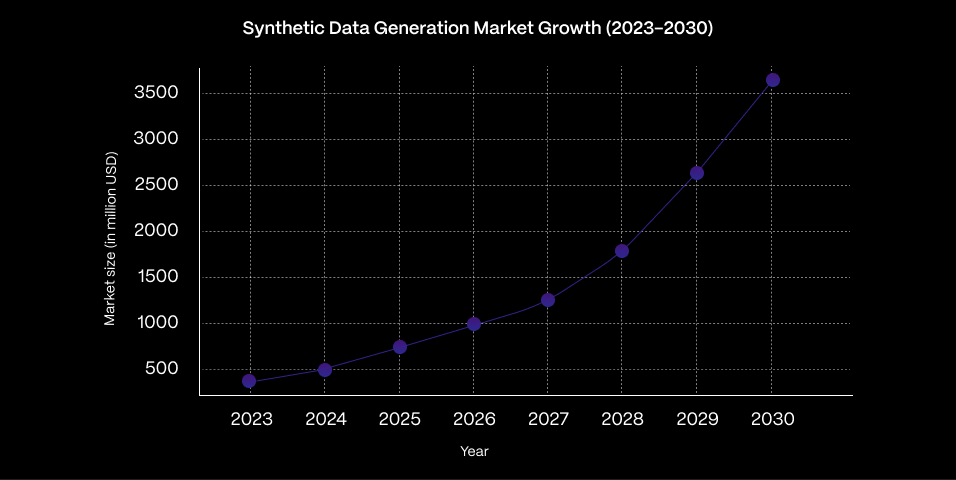
By combining generative AI models (like GANs and diffusion models) with data pipelines, organizations can generate synthetic AI data to train and validate their systems at scale. In short, synthetic AI and generative AI synthetic data are unlocking new possibilities across industries – from preserving patient privacy in healthcare to stress-testing logistics networks and optimizing smart manufacturing lines.
This blog post explores how synthetic data generation using generative AI transforms healthcare, logistics, and manufacturing, and why AI-driven businesses are rapidly adopting these capabilities.
What Is Synthetic Data (and Why Generative AI)?
Synthetic data are artificially generated datasets that mimic the statistical properties of real data without exposing sensitive information. In practice, generative AI models (such as GANs or advanced LLMs) learn patterns from real-world data and create new “data twins” on demand. This approach has several business advantages:
- Data Privacy & Compliance: Synthetic datasets eliminate personally identifiable information (PII), so companies can share and analyze data without risking privacy. For example, synthetic patient records preserve health patterns while stripping identity. This addresses strict regulations like HIPAA or GDPR. Notably, healthcare data breaches have been extremely costly, averaging about $19.93 million per breach in 2023, so using AI synthetic data can greatly reduce legal and financial risk.
- Data Scarcity: Many AI applications suffer from limited data (rare events, edge cases, new products). Synthetic data generation with generative AI fills those gaps. Generative models can simulate hundreds of realistic objects, scenarios, or event variations. This “filler” data boosts model accuracy. For example, one study showed that augmenting a medical image dataset with synthetic chest X-rays (via an AI diffusion model) measurably improved disease classification accuracy.
- Cost and Scalability: Real data collection (labeling, sensor deployment, security) is costly and slow. Synthetic data for machine learning online can be generated faster and cheaper. As one industry report notes, synthetic data provides a “controlled and scalable data source” for robust AI development. Companies like Nvidia and Databricks now offer tools (Omniverse Replicator, Unity Catalog) to automate synthetic data pipelines. Gartner predicts a surge in synthetic usage – by 2024, roughly 60% of data used to train AI platforms will be synthetic (up from 1% in 2021).
In sum, synthetic data generation using generative AI is one of the most exciting AI business applications today. It enables organizations to simulate new products, protect sensitive information, and accelerate AI model development. In the sections below, we examine how this plays out in key industries: healthcare, logistics, and smart manufacturing.
Healthcare: Privacy-Preserving Data and Better Models
Healthcare’s Synthetic Data Advantage
Patient data is sensitive, and sharing it is a legal maze. Synthetic data offers a safe workaround—replicating clinical patterns without exposing real identities.
- Privacy, Protected: Synthetic health records preserve patterns, not PII—helping meet HIPAA/GDPR standards and slashing breach risks that can cost up to $ 20 M.
- Data Amplification: For rare diseases and niche studies, generative AI scales small datasets. Synthetic X-rays and GPT-4-generated clinical notes have already improved diagnostic and NLP accuracy.
- Faster R&D: Pharma and hospitals can simulate trials or model patient cohorts instantly. Siemens, via Databricks, cut model training time from weeks to days using synthetic imaging.
- Ethical Innovation: From AI testing to patient digital twins, synthetic data powers safe, scalable breakthroughs—without the ethical landmines of real-world trials.
These benefits align with industry trends. As one review notes, synthetic data “opens new avenues for model training for diseases and simulation, enhancing research capabilities and improving predictive accuracy” in healthcare. In practice, hospitals, medtech firms, and health data startups report shorter development cycles and more robust predictive models by incorporating synthetic datasets.
Infographic idea: an illustrated chart could show the growth of synthetic data use in healthcare – e.g., rising from 1% of AI training data in 2021 to a projected 60% in 2024, alongside examples (synthetic patient charts, MRI scans).
Logistics: Simulating Supply Chains and Mitigating Risk
Generative AI is transforming logistics by creating synthetic data to simulate supply chains and test strategies, without disrupting real-world operations.
- Digital Twins & Scenario Testing: Companies are building virtual models of warehouses, routes, and inventories. Generative AI feeds these twins with synthetic scenarios, like demand spikes or port shutdowns, to test resilience ahead of time.
- Risk & Contingency Planning: AI-trained on synthetic “what-if” events helps planners assess risk and optimize routes, even for rare disruptions like factory fires or extreme weather. UPS is already using this to improve delivery networks.
- Forecasting Smarter: Synthetic customer data boosts demand forecasting for product launches or holiday seasons, allowing better stock decisions before real data exists.
- AI Model Training: Autonomous warehouses use synthetic simulations to train robots and vision models safely, before a single package moves in the real world.
The business impact is significant. Digital-twin simulations powered by synthetic data can reveal bottlenecks and guide investment. Ultimately, logistics leaders see generative AI synthetic data as a force multiplier: faster insights, lower rework, and robust supply chains.
Smart Manufacturing: AI-Powered Quality, Uptime & Innovation
Generative AI and synthetic data are transforming Industry 4.0—boosting precision, uptime, and agility:
- Quality Control: AI vision systems struggle with rare defects. Synthetic data solves this by generating lifelike defect images (e.g., rust, cracks) from CAD models. Agmanic Vision trained models to detect unseen faults using only synthetic welded-part images. Databricks confirms: this approach catches more defects before shipping.
- Quality Control: AI vision systems struggle with rare defects. Synthetic data solves this by generating lifelike defect images (e.g., rust, cracks) from CAD models. Agmanic Vision trained models to detect unseen faults using only synthetic welded-part images. Databricks confirms: this approach catches more defects before shipping.
- Predictive Maintenance: Simulated sensor data helps AI spot failures before they happen. McKinsey highlights digital twins enhanced with synthetic logs; Deloitte reports 25% more productivity and 70% fewer breakdowns. Bonus: Smaller manufacturers can now compete without mountains of real data.
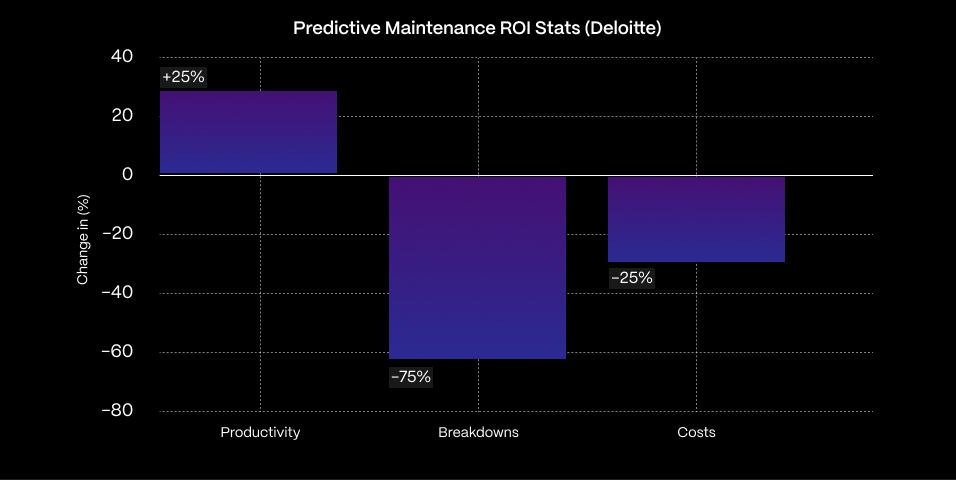
- Robotics & Automation: AI-trained bots need consistent vision. Generative tools (like NVIDIA’s TAO) simulate varied lighting and surfaces, so robot eyes don’t blink, even when shifts do.
Overall, smart manufacturing becomes more adaptable. An industry blog summarizes: generative AI enables manufacturers to train a broader variety of vision models and catch “rare defects with data that was previously too sparse”. In practice, this means higher yields, less waste, and faster time-to-market. Generative AI synthetic data is essentially the new raw material for Industry 4.0: factories that simulate themselves, learn continuously, and optimize at scale.
Industry-Wide Trends in Synthetic AI
Embedded into ML Pipelines:
Generative AI is now a tunable input, balancing data diversity and realism to reduce cost and boost model performance.
On-Demand Access:
Synthetic data for machine learning is now available online—cloud platforms let teams generate data with a few clicks.
Key Challenges | Best Practices |
---|---|
Ensuring realism & fairness | Rigorous QA process |
Avoiding biased or flawed simulations | Ethical oversight & audits |
Preventing reverse engineering of sensitive data | “Chain of custody” for synthetic datasets |
Big Picture:
Synthetic data is fast becoming a standard in AI business applications, from automation to personalization.
Closing Insights
Generative AI-powered synthetic data generation is no longer a niche R&D project – it’s a proven business tool. In healthcare, it is accelerating drug discovery and personalized medicine; in logistics, it is turning complex supply chains into adaptive, self-learning networks; and in manufacturing, it is driving the shift to smart factories.
As Gartner and industry analysts predict,
“Synthetic data will soon form the majority of AI training inputs, fundamentally changing how data-driven businesses operate.”
For business leaders, the message is clear: invest in synthetic data solutions now to stay ahead of the curve. Integrating synthetic data generation with generative AI into your AI roadmap can unlock faster innovation and stronger risk management. As we have seen, real-world case studies span from Siemens Healthineers cutting development time by 80% to UPS simulating its entire delivery network. The potential is vast.
In conclusion, synthetic data represents a transformative AI business application. By leveraging generative AI synthetic data, enterprises across sectors can train better models, protect privacy, and explore future scenarios – all at unprecedented speed. For more on generative AI’s broader impact on businesses, see our case study in Generative AI Transforming Healthcare Businesses. The future of industry is synthetic, and the companies that harness it will lead the market.
FAQs
1. What is synthetic data generation with generative AI?
Synthetic data generation with generative AI involves using machine learning models, such as GANs or diffusion models, to create artificial data that mimics real-world data. This technique is widely used to train AI models where real data is scarce, sensitive, or expensive to acquire.
2. How is synthetic data used in AI business applications?
Synthetic data is powering a range of AI business applications—from improving computer vision in manufacturing to enhancing predictive analytics in logistics and safeguarding patient data in healthcare. It allows businesses to safely and cost-effectively train models without relying solely on real-world datasets.
3. Why is synthetic data important for machine learning online?
Synthetic data for machine learning online enables companies to access ready-to-use, tailored datasets via cloud platforms. This democratizes AI development, letting even small businesses build powerful models without investing in complex data collection or labeling pipelines.
4. How does synthetic AI help in smart manufacturing?
In smart manufacturing, synthetic AI supports quality control, predictive maintenance, and robotics training. It creates realistic simulations of rare defects or machine failures, helping AI systems learn faster and perform more reliably on the factory floor.
5. Is synthetic data safe to use in healthcare and other regulated industries?
Yes—when done right. Generative AI synthetic data can protect privacy by removing personally identifiable information. However, organizations must implement strict validation, ethical checks, and data governance to avoid bias and prevent re-identification.
6. What industries are using synthetic data for AI right now?
Synthetic data is being used across several sectors—particularly in healthcare, logistics, and manufacturing. These industries benefit from improved model accuracy, faster deployment, and fewer data privacy concerns, thanks to synthetic data generation using generative AI.
7. What’s the difference between AI synthetic data and traditional data augmentation?
Traditional data augmentation tweaks existing data (like rotating images), while AI synthetic data is generated from scratch using models trained to replicate real-world patterns. Synthetic data offers greater diversity and scalability, especially for complex AI training scenarios.