AI-Driven Predictive Analytics in Logistics
The global supply chain is transforming, with predictive analytics at the forefront of this evolution. Businesses leverage data to anticipate future challenges, optimize operations, and gain a competitive edge. Let’s explore how predictive analytics reshapes supply chains, backed by relevant statistics and practical insights.
Understanding Predictive Analytics in Supply Chain
Predictive analytics involves statistical algorithms, machine learning, and data mining techniques to analyze historical and real-time data. Supply chain management helps businesses forecast demand, identify risks, optimize inventory, and improve delivery timelines.
Key Benefits:
1. Demand Forecasting: Predicting customer demand to prevent overstocking or stockouts.
2. Risk Mitigation: Anticipating disruptions like weather events or geopolitical issues.
3. Cost Reduction: Streamlining operations to minimize unnecessary expenditures.
4. Improved Decision-Making: Offering actionable insights for strategic planning.
Applications of Predictive Analytics in Supply Chains
1. Demand Forecasting
Accurate demand forecasting is key to an efficient supply chain, and predictive analytics makes it possible by combining sales data, market trends, and external factors. Amazon uses this approach to predict purchases and pre-ship items, cutting delivery times. According to a report, predictive analytics boosts inventory accuracy to 95% and reduces carrying costs by 20%.
2. Risk Management
Supply chains face risks like natural disasters, supplier disruptions, and economic instability. Predictive analytics leverages real-time data to identify potential risks and recommend mitigation strategies. For instance, Maersk uses predictive analytics to anticipate weather disruptions, enabling route adjustments that save millions annually. Notably, 73% of companies believe predictive analytics effectively manages supply chain risks, according to Deloitte’s Global Supply Chain Survey.
3. Inventory Optimization
Balancing inventory levels is essential to prevent understocking or overstocking, and predictive analytics plays a key role in achieving this. By analyzing demand patterns, seasonal trends, and lead times, businesses can optimize inventory management. For instance, Walmart leverages predictive systems to analyze sales data and streamline restocking processes. According to McKinsey & Company, predictive analytics can reduce inventory holding costs by up to 25%, making it a powerful tool for operational efficiency.
Related Article: Applications of AI in Business
Challenges in Implementing Predictive Analytics
Despite its advantages, implementing predictive analytics in supply chains comes with challenges:
- Data Quality: Inaccurate or incomplete data can skew predictions.
- Integration Issues: Merging predictive analytics with existing systems requires significant investment.
- Expertise Gap: Skilled personnel are needed to manage complex algorithms and insights.
- Initial Costs: The upfront cost of predictive systems can deter smaller businesses.
Steps to Implement Predictive Analytics in Your Supply Chain
1. Assess Current Systems: Evaluate existing data collection and management processes.
2. Invest in Technology: Choose tools like SAP Analytics Cloud, IBM Watson, or Tableau.
3. Train Teams: Develop internal expertise or hire skilled professionals.
4. Pilot Programs: Test predictive models in small-scale projects before full deployment.
5. Monitor and Improve: Continuously refine models based on feedback and performance.
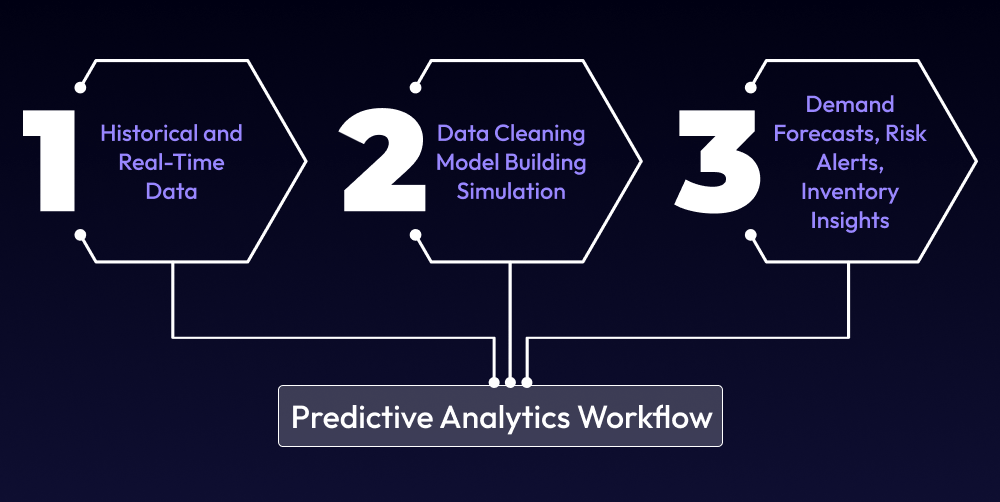
Case Study: Predictive Analytics in Action
Company: Procter & Gamble (P&G)
P&G implemented predictive analytics to optimize its supply chain. By analyzing historical sales, social media trends, and economic indicators, they accurately forecasted product demand. This approach:
- Reduced inventory costs by 15%
- Improved delivery timelines by 20%
- Increased customer satisfaction ratings
Source: Harvard Business Review
The Future of Predictive Analytics in Supply Chains
As technology advances, predictive analytics will evolve with:
- AI Integration: Enhancing model accuracy and speed.
- IoT Expansion: Providing real-time data from connected devices.
- Blockchain Synergy: Ensuring data transparency and security.
Statistic: The predictive analytics market in supply chain management is projected to grow at a CAGR of 21.3% from 2023 to 2030 (Grand View Research).
Conclusion
Predictive analytics is no longer a luxury but a necessity for modern supply chains. By leveraging data-driven insights, businesses can enhance efficiency, mitigate risks, and stay ahead in a competitive landscape. The journey may have challenges, but the rewards far outweigh the efforts.
FAQs
1. What is predictive analytics in supply chain management?
Predictive analytics uses historical and real-time data to forecast future trends and optimize supply chain processes.
2. How does predictive analytics improve demand forecasting?
By analyzing sales data, market trends, and external factors, predictive analytics provides accurate demand projections, preventing overstocking and stockouts.
3. What tools are used for predictive analytics in supply chains?
Popular tools include SAP Analytics Cloud, IBM Watson, Tableau, and custom machine learning models.
4. What industries benefit most from predictive analytics in supply chains?
Industries like retail, manufacturing, logistics, and healthcare benefit significantly from predictive analytics.
5. What are the main challenges in adopting predictive analytics?
Key challenges include data quality issues, integration difficulties, high initial costs, and a shortage of skilled personnel.