Gen AI in Mental Health – Use Cases & Solutions
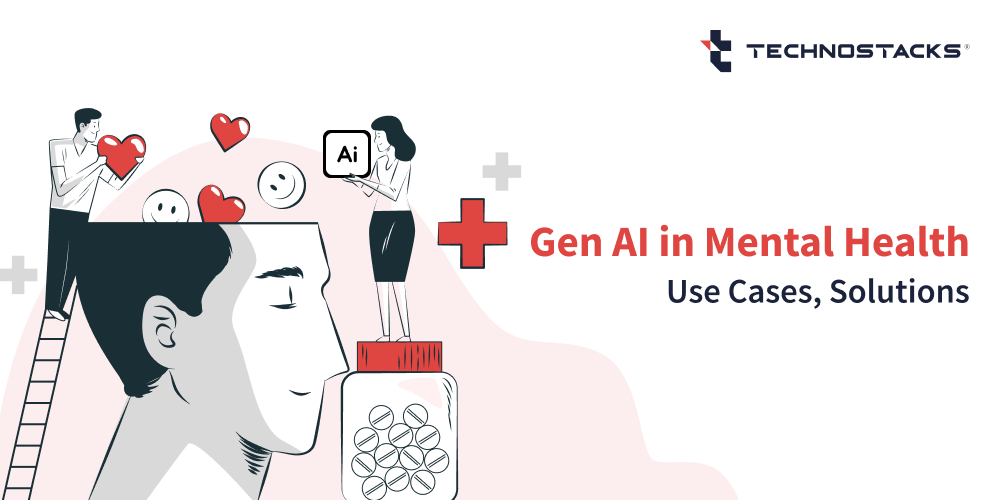

Technostacks
Artificial intelligence is developing fast and disrupting every industry. It is reasonable that mental health will be its next frontier. AI is not going to replace traditional therapy anytime soon, but its potential in mental healthcare is vast.
What is Generative AI?
Generative AI is a type of AI that generates new text, visual, and audio content. It has been simplified and made accessible to the average user. Anyone can use generative AI to massively speed up content creation tasks.
Based on that premise, that is captured or curated from the existing data using machine learning algorithms, identifies its pattern, and generates output related to the dataset provided. Such advanced pattern modeling enables it to predict, simulate, and create content with immense potential in mental health care.
Generative AI in Mental Health
Generative AI will afford the industry of mental health care the ability to innovate many facets of traditional therapy; this includes psychological counseling services to more proficient diagnoses and treatment plans. It is designed to duplicate the personal touch of manual therapists while at the same time taking full advantage of its remarkable abilities in data processing and analysis.
For example, it can be used for more efficient diagnostic testing and to create personalized treatment plans. It can also be used for natural language processing (NLP) applications, such as identifying speech patterns in real time or generating summaries of conversations between therapists and patients.
The best example of a Gen AI-powered tool for mental health therapy is XAIA: the Extended-Reality Artificially Intelligent Ally, which uses immersive virtual reality and generative artificial intelligence to offer self-administered and timely mental health support.
In addition, generative AI can enable the personalization of mental health care, providing therapeutic interaction, supporting diagnosis, and monitoring mental health.
According to Statista, worldwide adoption of GenAI for healthcare is represented below in all the use cases.
A Summary of Use Cases
Summarizing ways generative AI can be used in a mental health context:
- Personalized Mental Health Interventions: Generative AI can be used to construct personalized mental health interventions. From data analysis about a patient’s behavior, emotions, and state of mind, a generative model may recommend what he or she needs.
- Virtual Therapists: Generative AI can also serve purposes for virtual therapists, where cognitive behavioral therapy (CBT) is administered or some other type of therapy. Programs of this thread for AI therapists could be available 24/7 for support when human therapists would not be available.
- Mental Health Monitoring: Generative AI can monitor a patient’s mental health over time. It can analyze data from multiple sources, including but not limited to social media posts, data gathered from wearable devices, and self-reported mood scores that indicate the state of a patient’s mind.
- Generating Hypotheses for Research: Generative AI has possibilities for hypothesis formulation in mental health research. Such large-scale analysis of datasets enables these models to identify patterns and correlations that might go unnoticed by human researchers.
- Simulating Mental Health Conditions: Generative models can simulate mental health conditions that can be utilized for training healthcare professionals or testing interventions in a controlled environment.
According to the Healthcare professionals
“ChatGPT may be best suited for therapy modalities that are minimally dependent on emotional/supportive statements or the interpersonal relationship between patient and therapist.”
Recognizing the Limitations and Addressing the Challenges
1. Patient Data Privacy
Data privacy is a major concern in GenAI-assisted healthcare, as AI models are trained using large volumes of patient records. Any unauthorized access could potentially impose a very high-security risk for the users.
Patients are not forthcoming to reveal information about mental health owing to a personal stigma about their character. This challenge is expected to be one of the reasons that would deter user interactions for mental wellness-related queries through AI chatbots.
To resolve this, data can be anonymized so that, if leaked, the data will be useless.
Related Article: AI in Healthcare
2. Adversarial Attacks
Although AI might be programmed to respond no differently from human-like reactions, it equally has tendencies to be susceptible to adversarial attacks.
Hackers may manipulate AI systems to make inaccurate diagnoses. Therefore, self-adaptation techniques in model training are essential in enabling models to detect any pattern uncommon in the data.
3. Data Sharing
It is very critical to securely transfer health records so that unauthorized persons can’t access the data or manipulate it. GenAI models deal with a lot of data for training them, so keeping the data source authentic is very essential.
Various cryptography algorithms, such as RSA and ECDSA, can enable data encryption, which will prevent the health record from getting jeopardized.
4. Legal Compliance
Mental health-related GenAI systems have to follow legislation such as HIPAA and GDPR. HIPPA and GDPR include some strict cybersecurity measures that ensure patients’ privacy.
Encryption techniques, access restriction, and safe storage are some of the ways used to ensure anonymity and safety for patients. This legally builds trust between a patient and a medical professional.
5. Bias
The biases of the data on which they are trained make GenAI algorithms prone to them. These inconsistencies may lead to the unequal treatment of various people from different origins and thus further increase existing inequalities in health care.
The main demand will be linked with developing relevant mechanisms that will allow detection bias and its internal handling within genAI systems correcting it, and finally providing unbiased outputs. In this way, fairness and objectivity of diagnosis would be guaranteed, boosting patient confidence.
Related Article: Benefits of AI in Healthcare
6. Authentication
GenAI-based models need large amounts of data to train the model in the best possible manner; hence, data should be accessed by authenticated users only.
To prevent this risk, behavior-based biometric authentication can be done using various ML and cryptography techniques so that the results are error-free.
7. Patient-Data Integrity
Trust needs to be developed with patients who depend on GenAI tools for seeking suggestions for their mental health. If the integrity of data is not maintained, loyalty will be affected. The anomaly detection algorithms find unusual patterns in health data and prevent wrong diagnoses further.
Key Takeaway
Summarizing the entire consent of the role of GenAI in mental health, it offers considerable value in psychological health; its implementation has a certain set of problems associated with it, and these are being looked after and considered with due care.
This is an era of modernized research and development, with ongoing and future developments apt to help build on and navigate the path for the solutions to these thrown-up challenges.
FAQs
1. How can AI be used to improve mental health?
AI might monitor online activities and usage of social media regarding early detection of cyberbullying, anxiety, and depression in a youngster. Early intervention facilitated by AI will help in the development of healthy emotional coping mechanisms before mental health problems escalate among young users.
2. How is generative AI used in mental health?
Behavioral Health and AI-based Image Creation
Examples include decreased emotional distress, improved patient education and learning, reduced health disparities and stigma, enhanced interaction among patients and providers, as well as increased personalization of treatment and access to care.
3. What is the generative AI use case for healthcare?
Data Analysis and Interpretation: Gen AI analyses complex data from both genetic and molecular levels, further helping health professionals interpret information related to individualized treatment plans. This will be able to identify specific genetic markers and analyze what those mean regarding personalized care.
4. How does AI help solve problems?
AI can analyze huge amounts of data to comprehend trends, patterns, and anomalies that are difficult for the human eye to see. This ranges from finding fraudulent activities in finance to early warnings for outbreaks of diseases in healthcare. AI can make predictions based on events likely to happen in the future.
5. How to solve AI bias in healthcare?
Here are 3 ways in which to mitigate AI bias:
1. Research and development. Building models and collecting data should be representative of the population they’re trying to address.
2. Data collection.
3. Algorithm development & application.