How Machine Learning Can Detect Fraud In Banking & Finance Sector?
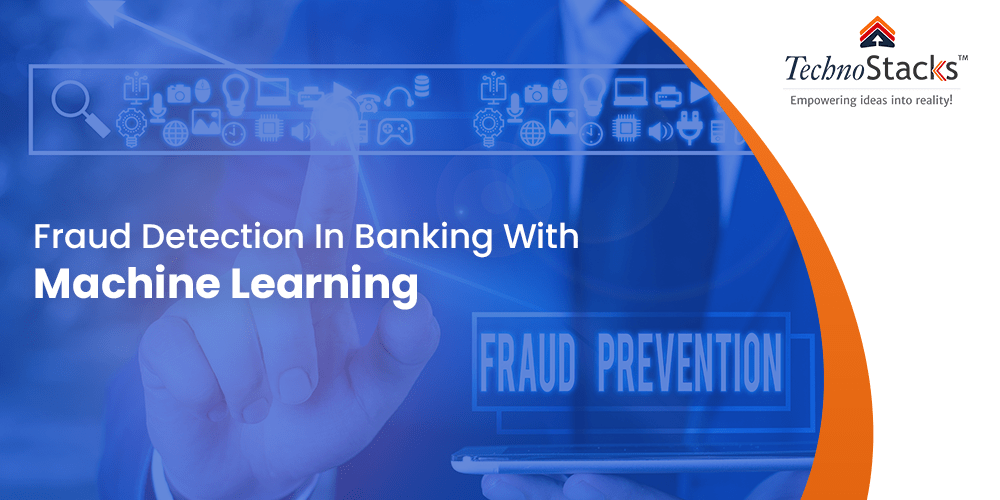

Technostacks
Artificial Intelligence and machine learning- this technology have a significant impact on almost every field, and now it is helping the financial sector to go through one of the most significant transformations ever. The financial industry is becoming pretty advanced every day, and there have been a lot of changes lately. With the help of technology, the tasks that employees usually take some days to complete are done within some seconds. And this indeed is some severe transformation.
However, technology has also resulted in an increased number of frauds in the banking sector. Now, everything that a person needs regarding their bank details is available on their phones and their laptops. This scenario makes it easy for hackers to get into the device and steal the amount. Vast amounts of money are at stake with these kinds of frauds, and people are going through a lot of stress just because of it. This particular case is where machine learning is going to step in.
This technology can detect frauds and protect customers from potential dangers. It is common to have a question popped in your brain- how machine learning in finance and banking helps in scam detection? This article is going to discuss the same. You will get to know everything you need to know about fraud detection and what you can do to handle it.
Fraud Occurrences and Statistics
The cybercriminals are getting smarter every day. And they are leveraging the same technology that has been helping customers for their advantage. The banks and other financial institutions have no other option rather than employing more advanced tactics to curb the frauds. The business firms are spending more and more money with every passing year so that they can control these kinds of fraud. While it is helping in some instances, it is not as effective, and people are still falling prey for various types of fraud from time to time.
While there are various ways to fall prey for fraud detection, one of the most common issues is credit and debit card fraud. With the increase in eCommerce shopping and online money transfers, the number of people who are falling prey for fraud is increasing with every passing day.
According to Statista, the online fraud amount that has been lost by Americans in 2015 is 1.1 billion USD, and it reaches 3.5 billion USD by 2025. More than 82% of companies have fallen prey to this card fraud, and the amount of loss is increasing with every year passing by.
The direct cost frauds are also impacting companies as they lose sales when genuine transactions are declined because of fraud management. The companies now have a more significant task before them, and they have to differentiate between legitimate and fraudulent transactions without affecting customer experience in any way.
Digital Solution For Online Fraud Detection In Banking
Bankers approached this issue manually using a set of rules. This case means that they will use a complex set of criteria for suspicious flag transactions that need to be reviewed manually.
This process is useful to discover fraud detection in the banking sector, but it is not something that you can rely on. It won’t detect the frauds, and this has become another excellent chance for cybercriminals to make use of it. The banks and other financial institutions now have to get ahead of the criminals and use advanced options like applications of machine learning in finance and banking.
How Machine Learning Helps Fraud Detection in the Banking Sector?
Banks have vast volumes of customers’ data, and this is something that will help them understand and analyze the patterns. The financial sector can apply the same data and use machine learning to flag any kind of fraudulent transactions with ease. They check for any type of inappropriate behavioral patterns and file them immediately. This scenario will help them in keeping things under check without the requirement of human intervention.
Machine learning is always a step ahead when compared to the cybercriminals, and it keeps an eye on any kind of newly adapted technique by the fraudsters. As the accuracy of machine learning increases, it becomes capable of identifying the false and incorrect flagging too. There can be some genuine incidents which are flagged because of some mistake, and the machine learning can identify them also and ensures that these kinds of errors won’t happen again.
Now, the firms will have a chance to mitigate their financial losses and protect their reputation as well without causing any kind of problem for customers. It improves customer confidence in the organization, which is something that they need.
How ML-Based Fraud Detection Works?
Earlier, you have understood the leading theory behind why to implement machine learning. Now, you are going to see how this machine learning-based fraud detection works and the process of fraud detection with machine learning.
Cybercriminals are relatively quick, and there is no other option for the bank rather than going with this machine learning. There are two types of fraud detection machine learning models which are known as –
- Supervised machine learning
The supervised machine learning uses the already provided data by human beings during their detection and finds out similar patterns. It learns the intricate data patterns in the sets provided by the business and uses it for the further exposure of any kind of fraud. These are the methods for fraud detection using machine learning.
- Unsupervised machine learning
This unsupervised machine learning, just like its name, uses the datasets that are not labeled, and it infers the inner data structure by itself.
Types Of Machine Learning Algorithms Used in Fraud Detection
Machine learning is a technique that adapts different types of algorithms to ensure that fraud detection is identified. It hugely depends upon the data provided by banks. Here is the list of algorithms that Machine Learning follows –
- Logistic Regression
This is a model that checks the relationship between different variables in the data sets. This scenario can come in use to create an algorithm that will predict whether a particular transaction is good or bad.
- Decision Trees
The decision trees come in use to create a particular set of rules that will help machine learning in identifying normal behavior. This scenario even helps with the training.
- Random Forests
The random forests are an ensemble of decision trees. Multiple trees are classified and put under one robust classifier for better understanding.
- Neural Network
Similar to its name, this algorithm depends on the working of a human brain. The neural networks are pretty powerful, and they can identify the fraud in real-time.
All of the above algorithms come in use in a supervised technique. In the unsupervised method, there will be clustered algorithms that will group all the similar data points together. They are mainly used for anomaly detection.
- K-Means Clustering
This algorithm divides a dataset into different clusters. This algorithm works iteratively and assigns a different set of data points to a particular predefined number of classes. This case is dependent on the features present in a dataset.
- Local Outlier Factor
This is an algorithm that calculates the local density of data points and allows in identifying the regions with similar frequency. This locality concept will help in identifying the locality points that will distinguish points with much lower density.
- One-Class SVM
This learns a function that has been used in novelty detection. It detects the incidents that happened rarely and will notify immediately.
So this is how machine learning applications can help in banking and fintech. Machine learning is pretty advanced, but it still has a set of limitations. The process of fraud detecting with machine learning is useful when the right set of data is provided only. Machine learning feeds on the data only, and if the proper amount of information is not provided to it, it won’t work as required. There are various kinds of approaches that help in dealing with this problem.
Financial firms are overcoming this particular issue with the help of creating artificial data points that usually replace the original ones. They are using techniques like oversampling and undersampling. These techniques assist in developing data that will help in restoring original data.
Banks and financial institutes are using every method possible in order to create data that will help them with the research. The first stage here is automated, but the second stage process requires manual checking. This scenario may result in different kinds of human errors, and this is the reason why organizations should not use this process and adapt to a new and reliable one.
Key Takeaways
Cybercriminals are increasing with every day passing by, and they are participating in various kinds of fraudulent acts too. It has become essential for financial organizations and banks to implement machine learning to curb such a variety of issues. With banks and financial institutions, there is huge money at stake, and banks have to ensure that they provide proper safety to their customers and ensure that their money is safe.
If you are someone who wants machine learning-based apps or solutions, then get in touch with us. We at Technostacks, have been dealing with machine learning and AI app development for a long time and can provide tailored services depending upon your requirement.