ArtificiaI Intelligence in Medical Diagnostics
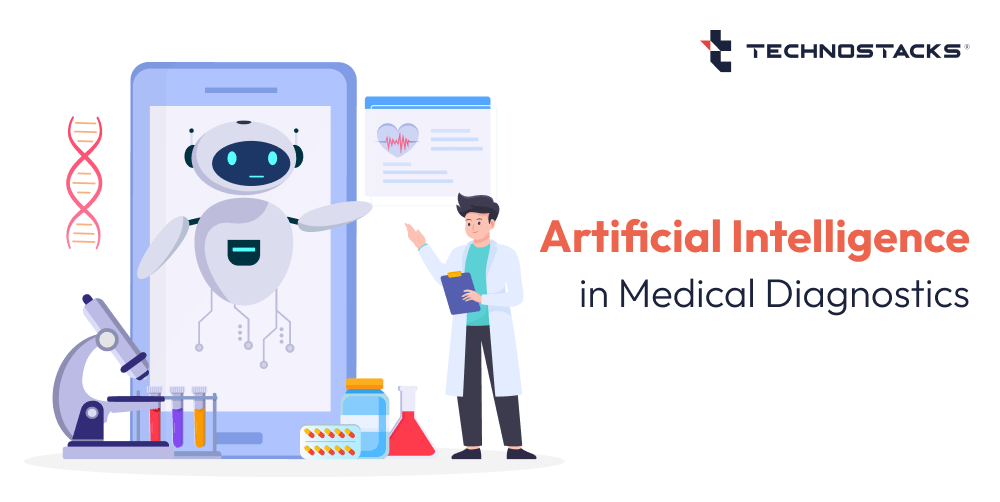

Technostacks
Accurate diagnosis in medicine forms the backbone of healthcare treatment. On-time treatment ensures better treatment outcomes and helps drastically reduce costs, among many other benefits.
However, with traditional diagnostic practices, limitations in human perception, resource constraints, and the complexity of medical data often result in inefficiency. In such a background, AI has emerged as the transformative force dramatically changing how diagnoses will be done.
AI technologies have immense potential for increasing precision, simplifying workflows, and reducing errors in medical diagnosis. Let’s explore the changing face of diagnostics with AI, underpinned by real-world applications, trending statistics, and a view toward future possibilities.
What is AI in Healthcare?
AI in healthcare represents a set of algorithms, machine learning models applied to advanced computing techniques while analyzing complex data in medical science. Basically, unlike classic tools and techniques, it can manage a lot of data in finding meaningful patterns and making predictions using them with high precision.
Key AI technologies in diagnostics include:
- Machine Learning (ML): Enables predictive modeling and decision-making.
- Natural Language Processing (NLP): Extracts insights from clinical notes and medical literature.
- Computer Vision: Analyzes medical images for patterns and anomalies.
Applications in Diagnostics
AI is being deployed in a variety of diagnostic processes, including:
- Disease Detection: Areas in which AI tools particularly excel include early detection based on imaging and pattern recognition of diseases like cancer, conditions of the heart, and neurological disorders.
- Personalized Medicine: AI analyzes genetic and clinical data to recommend personalized treatments.
- Predictive Analytics: Forecasts disease course and complications that may occur.
- Imaging and Pathology Interpretation: AI enhances the analysis of X-rays, CT scans, and MRIs, outperforming human specialists in many cases.
How AI Tools Improve Diagnostics
Enhancing Accuracy
AI minimizes the margin of error by eliminating human subjectivity. For instance, Google’s DeepMind has achieved a 94.5% accuracy rate in diagnosing eye diseases, surpassing human specialists. AI’s ability to analyze large volumes of data helps uncover subtle patterns that might go unnoticed by clinicians.
Improving Efficiency
AI lessens the time taken up by diagnosis processes. Indeed, it could be done in minutes using automated tools for thousands of images or test results and release health professionals to take care of patients.
Pattern Recognition
AI is very good at pattern recognition in medical data, even for very rare conditions. For example, Face2Gene, a tool that leverages facial recognition algorithms to diagnose genetic disorders, can highlight conditions that may take years to diagnose by a physician.
Reducing Error Rates in Healthcare
Common Diagnostic Errors
The results can be quite serious about diagnostic errors. Misdiagnoses, overdiagnoses, and delayed diagnoses are associated with high percentages of negative patient outcomes. It is estimated that 10% of all patient deaths globally were due to diagnosis errors according to a study in 2024.
How AI Reduces Diagnostic Errors in Healthcare
1. AI-Assisted Decision Support Systems
- Clinical Decision Support (CDS):
- AI in Radiology:
- Efficiency Gains:
- Streamlining Workflow:
The AI would analyze the patient data and then provide recommendations, based on that, for diagnosis and treatment, much like a guideline. Tools serve to reduce diagnostic errors and also increase the speed and precision in decision-making.
Aids in diagnosing diseases such as pneumonia, tuberculosis, and brain tumors. In a study of 2023, JAMA Network Open showed that AI reduced diagnostic errors by 18%.
2. Reducing Cognitive Load
Automates routine tasks like image analysis and data entry, freeing time for complex care.
AI reduces bottlenecks with automated triage, image sorting, and medical record review, enhancing healthcare efficiency.
Benefits of AI in Medical Diagnostics
Improved Patient Outcomes
AI presents the possibility of early diagnosis of diseases, thus giving much-needed timely interventions. For example, a study showed that AI tools increased breast cancer diagnosis by 12%, thus saving thousands of lives a year.
Cost-Effectiveness
AI lowers healthcare costs by managing and simplifying diagnosis workflows to facilitate early treatment. Early diagnoses can prevent expensive complications that raise readmission cases in hospitals.
Increased Accessibility
AI-powered diagnostic tools bring healthcare to underserved regions through mobile applications and telemedicine platforms. For instance, mobile-based AI applications can enable rural health workers to diagnose diseases such as tuberculosis and malaria using meager resources.
Related Article: Benefits of AI in Healthcare
AI in Medical Diagnostics
AI revolutionizes X-ray, computed tomography scans, and MRI interpretations with very high precision, surpassing human capabilities in the detection of diseases related to cancer and disorders affecting vision.
The use of AI in anomaly detection related to cardiac and neurological conditions has significantly increased early disease detection. Predictive analytics enables early detection of cancer and heart diseases, thereby reducing fake positives and negatives to a greater extent.
AI eliminates human fatigue and bias, therefore minimizing errors, thus making healthcare delivery equitable and consistent. Capable of working 24/7, it boosts efficiency by enabling clinicians to make timely, accurate decisions for improved patient outcomes.
Limitations of AI in Medical Diagnostics
Data Privacy and Security
AI systems require immense patient data, raising several privacy and compliance issues. Laws such as HIPAA and GDPR impose strict protection for data, yet breaches still pose a looming threat.
Integration with Existing Systems
AI adoption in healthcare requires upgrading the infrastructure, training professionals to handle the systems, and integrating AI tools with the existing system, which may be costly and time-consuming.
Ethical Considerations
Bias in AI algorithms can lead to disparities in diagnosis and treatment. The application of unbiased and equitable AI is becoming an urgent issue.
Trending Statistics
The adoption of AI in medical diagnostics has seen exponential growth in recent years.
Key statistics include:
Market Growth: The global AI healthcare diagnostics market was estimated at around US$3.7 billion in 2023. This spending is expected to reach a high of US$8.6 billion by 2028, recording a CAGR of about 18.7 percent to a source insight.
Accuracy Rates: AI tools in imaging diagnostics have been able to achieve accuracy rates above 90% in detecting conditions such as lung nodules and breast cancer.
Adoption by Providers: In the survey conducted in 2024, 72% of healthcare providers reported plans for increased investment in AI-driven diagnostic tools over the coming five years.
Future Prospects of AI in Medical Diagnostics
Emerging Technologies
- Quantum Computing: The unparalleled power of computing will grant faster and more accurate diagnostics.
- AI in Genomics: The integration of AI with genomic data to predict hereditary diseases and recommend their prevention.
- Wearable AI Tools: Devices that can monitor one’s health in real-time and provide information on cardiovascular health, and blood sugar levels, among others.
- Global Standardization: Efforts to create universal protocols for AI diagnostics to ensure consistency across regions.
Trends to Watch
Vision for the Future – AI in Medical Diagnostics
Here are some trending pointers for “Vision for the Future”:
1. Precision Diagnostics: The New Standard
2. Personalized Patient Care
3. Revolutionizing Radiology and Pathology
4. Reducing Diagnostic Timeframes
5. Unleashing the Power of Big Data
6. Bridging Global Healthcare Gaps
7. Enhanced Workflow for Clinicians
8. Combating Diagnostic Challenges
9. Ethical AI Implementation
10. Collaborative Future: AI and Human Expertise
The Start of a New Era in Medicine
Early Stage of Integration:
Historical Parallels:
Future Potential:
Conclusion
AI is changing the face of medical diagnostics, standing out in unparalleled accuracy, efficiency, and access. The improvements in AI have solved several health challenges and will continue saving lives to move toward a smarter, quicker, and more inclusive future in health.
And that is just the beginning of this journey of AI in medical diagnostics, as the potential for more is unlimited. With this technology, a whole new era of precision medicine opens up to the healthcare industry, changing patient care for generations to come.
Let’s harness the power of AI to create a better, fairer world, contact us!
FAQs
1. How can AI improve diagnostic accuracy in healthcare?
AI algorithms enhance diagnosis by improving the analysis of complex medical data and reducing the likelihood of human error. Early Detection: Machine learning models can indicate the early stage of cancers or heart diseases and thus help in timely interventions.
2. How accurate is AI in diagnosing?
Physicians using AI to guide their diagnoses did better: Their diagnoses were about 81.1% sensitive and 86.1% specific. The improvement may seem small, but the differences are critical for people told they don’t have cancer, but do, or for those who do have cancer but are told they are healthy.
3. How accurate is AI in medical imaging?
A systematic review of the performance of these AI systems reported a pooled sensitivity of 84% and specificity of 61.5%.
4. How does AI improve accuracy?
AI analyzes information automatically and flags all inconsistencies or errors, allowing an organization to identify and correct them quickly. This improves data accuracy and reduces the occurrence of errors.
5. Can AI be 100% accurate?
AI works with probabilities and approximations: even with vast amounts of data and processing power available, certain outcomes are impossible to achieve, as artificial intelligence models are trained using historical data and only predict future behaviors based on uncovered patterns.